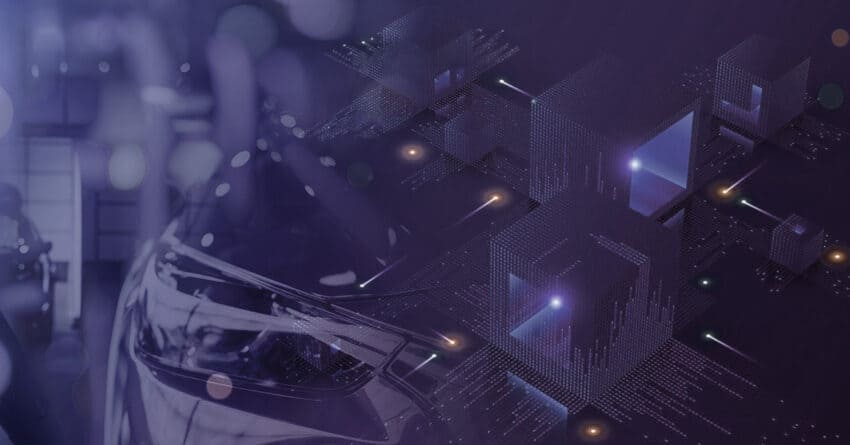
With thousands of dealerships and OEMs leveraging automotive AI today in some fashion, it’s clear that the industry has moved past the early adoption phase. The question surrounding Automotive AI has shifted from whether to use it to how to use it.
Researchers from Stanford University’s Institute for Human-Centered Artificial Intelligence just released the 2024 AI Index, packed with objective insights to help answer those questions – and many more – regarding AI. We’ve cut through hundreds of pages of research to highlight key findings every automotive retail leader should know when developing and executing their AI strategy, and expert tips on how to navigate each insight.
New AI findings most relevant to dealerships and OEMs:
#1: The data unequivocally shows that AI boosts employee productivity
The AI Index Report combined multiple productivity studies of customer support agents, consultants and more, and found that the use of AI has a profound impact on productivity. Workers leveraging AI not only deliver higher-quality work, but they do so in a much shorter time frame.
The uptick in quality and speed translates into financial gains as well. Another study cited in the report noted that 59% of surveyed organizations report revenue increases from implementing AI, and cost reductions have increased 10% since the previous year. These combined findings suggest AI is driving significant business efficiency gains.
Our take: We help thousands of dealers implement automotive AI and see similar results. When automotive retailers deploy AI at scale, they can drive sales, increase service revenue and boost customer lifetime value by enhancing the customer experience while increasing operational efficiency.
But what does that actually look like? In the automotive industry, AI works alongside dealership staff to ensure every lead and customer is engaged with 24/7 immediate, humanlike dialogue, and it continues the relationship once a buyer becomes a customer with personalized service outreach and on-demand campaigns. This frees staff members up to make more phone calls and deliver white-glove showroom and service experiences that translate into higher win rates and customer lifetime value.
#2: Yes, AI can beat humans on some tasks – but not nearly all
AI models made a significant breakthrough this year when they surpassed the human Massive Multitask Language Understanding (MMLU) benchmark. This metric assesses model performance in zero-shot or few-shot scenarios across 57 subjects, including the humanities, STEM, and social sciences. However, humans continue to outperform AI in significant critical areas, including mathematics, visual commonsense reasoning, and planning.
Our take: Achieving peak productivity isn’t about choosing humans or AI. It’s about creating a powerful team where dealership staff and AI work side by side in their respective areas of strength.
However, don’t confuse the need for humans and AI to work together with babysitting your AI solution. The best AI platforms can run independently, intelligently automating administrative tasks and routine conversations, but pulling in team members when most appropriate. With the proper tech stack integrations, AI will continuously communicate with your CRM, DMS, and inventory management platforms for a seamless customer experience. An experienced Automotive AI partner can also help you evaluate the best AI solution and implementation strategy for the unique needs of your organization.
#3: Closed Large Language Models (LLMs) perform better than open LLMs overall
It’s important to define the difference between open and closed Large Language Models before applying this insight to automotive retail. A closed LLM is accessible solely to its developers, whereas open models offer generally broad access, typically via public APIs.
The 2024 AI Index found that generally, closed models outperform open ones. Specifically, on ten selected benchmarks, closed models achieved a median performance advantage of 24.2%.
Our take: The Automotive AI landscape includes a continuum of solutions built on solely open models to fully closed models. Choosing a verticalized AI solution that is purpose-built for the automotive industry should be a top priority to ensure optimal business results. Next, retailers should prioritize AI solutions that combine the foundational strength of open models with the specialized training, industry-specific data, and safeguards that closed models offer against hallucinations, inaccuracies, and other poor outcomes.
Read our Automotive AI Buyer’s Checklist for more on choosing the right AI solution.
#4: AI investment has skyrocketed.
While overall private business investment declined last year, generative AI funding increased almost eightfold from 2022 to 2023, and nearly 80% of Fortune 500 companies discussed AI in their earnings calls in 2023.
Our take: These data points are leading indicators that AI will soon infuse nearly every consumer interaction, as it is quickly becoming embedded within the largest industries and organizations across the globe. For example, the four most dominant consumer technology providers in the world – Google, Meta, Amazon, and Microsoft – all have open-source AI models and have embedded AI deeply within their organizations. AI ubiquity will heighten the quality of consumer interactions, accelerate the overall pace of business, and enhance consumer and worker productivity. Dealers and OEMs that fail to embrace AI run the risk of being left behind.
#5: LLMs are poor self-correctors
Large language models (LLMs) face reasoning limitations that lead to hallucinations – a shortcoming researchers are keen to solve as AI adoption continues to surge.
Researchers from DeepMind and the University of Illinois tested whether an LLM could catch its own mistake without needing help – a concept called intrinsic self-correction. They found that when a model was left to correct itself, its performance declined across all tested benchmarks.
Our take: The threat of hallucinations is enough to scare some automotive leaders away from AI adoption altogether, but verticalized LLMs that are trained on industry-specific data can significantly reduce that risk. A sophisticated industry-specific LLM will continuously refine and adjust based on an ever-expanding set of consumer interactions and outcomes, customer feedback, and ongoing quality assurance reviews aimed at optimizing performance. Automotive AI vendors that take proper precautions against hallucinations and jailbreaks should be transparent about the methodology behind safeguarding dealers from reputational and business risks specific to the automotive industry. A solution with these precautions in place will protect your business and build confidence in team members as they begin working with AI.
Dealerships are historically built on labor-intensive business models, but AI presents a great opportunity to use that labor in more strategic and productive ways across the auto retail ecosystem. With a deeper understanding of how humans and AI can work together, now is the perfect time to embed or expand your use of automotive AI.