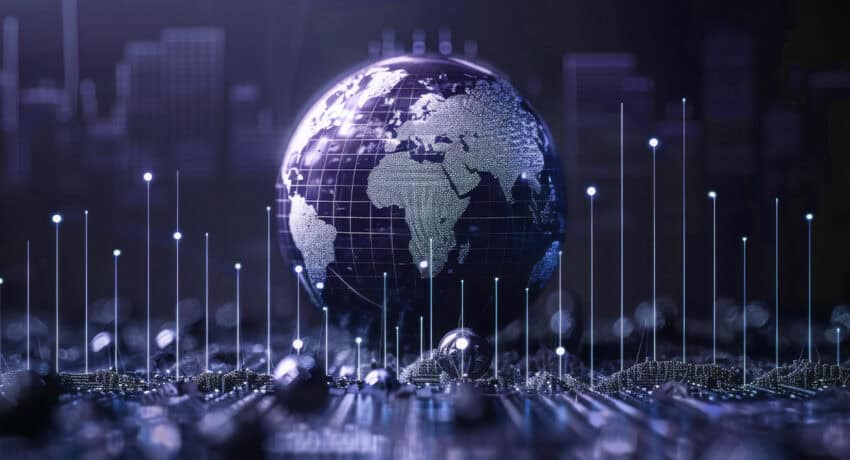
by: Michael Quigley, Co-Founder, Impel
Companies are choking while deploying GenAI. Leverage Vertical AI or die.
Writing in the Wall Street Journal in 2011, Marc Andresseen, noted venture capitalist and founder of Netscape, made the prophetic declaration that “software is eating the world.” He went on to portend the stratospheric successes that Google (Now Alphabet), Facebook (Now Meta), Amazon, Netflix, and LinkedIn would all go on to achieve. Today, amidst the hype cycle surrounding Generative AI, it is tempting to add a GenAI coda to Andressen’s declaration. However, when closely examining the value that GenAI has actually delivered thus far, it quickly becomes clear that many companies are still struggling to deploy GenAI in meaningful ways—indeed, many companies are practically choking on GenAI at present. In the end, I am an eternal optimist and believer that GenAI will eventually “eat the world.” Infrastructure GenAI companies such as OpenAI, Anthropic, Nvidia, et al— which are today’s media darlings— will indeed all likely go on to deliver massive value to consumers and shareholders alike. However, I predict that Vertical Generative AI companies, or Vertical AI companies for short, largely absent from the media hype cycle today, will go on to deliver an even more meaningful impact on the lives of consumers and business operations. Vertical AI is largely absent from the conversation today— and grossly underestimated at present.
GenAI deployments remain largely experimental today. Nearly half of companies in software, customer service, and marketing are exploring GenAI solutions. Yet less than 20% have successfully transitioned these initiatives into full production, and in more complex, high-stakes domains like legal and sales, adoption drops to below 10% (Bain). In an almost comical illustration of the matter, consulting giant Accenture now earns more revenue from advising companies how they might deploy generative AI, than OpenAI does on the technology itself (Accenture).
At Impel, we’re fortunate to rank among the world’s largest Vertical AI companies as measured by revenue, and we count fifteen Fortune 500 companies (automakers, auto retailers, and heavy equipment manufacturers) among our customers today. We’ve identified four key reasons why our customers have chosen to partner with us, instead of spending tens of millions of dollars on consultants in an attempt to deploy GenAI themselves. Our vertical AI platform has allowed our partners to outperform their peers in the market by successfully operationalizing GenAI in meaningful ways that have already generated significant ROI and business impact. We believe these four key reasons/ challenges are universally applicable beyond the global mobility market, and will lead to the creation of transformative applications parallel to Impel’s in every other vertical on the planet, from healthcare and real estate, to travel, dining, and more. Each industry or vertical use case represents a multi-deca billion dollar company in waiting.
Challenge #1: Infrastructure / LLM fine-tuning never ends.
As many a CTO has quipped, “software engineering never ends.” Products are never done: customers demand new features, edge cases and bugs must be addressed, and APIs require updating. And so it goes with Large Language Models (LLMs)— once they are deployed, the work has only just begun. In a world with an ever-shifting LLM leaderboard, remaining competitive requires running multiple infrastructure models at once across varying use cases in order to ensure that the right answer is being delivered against the right query at the right moment in time, for the lowest possible cost. For instance, at Impel, at any given time we are leveraging a proprietary mix of open-source LLMs in addition to our own specialized model— a custom LLM tailored to specific use cases and specialized for tasks in the mobility industry. Especially given today’s rapid rate of technological change, companies all too often fail to appreciate the ongoing engineering efforts required to customize and cost-optimize infrastructure LLM usage, and are thus not typically prepared to make the required investments.
Challenge #2: Siloed data.
LLMs are only as good as their training data. In order to deliver compelling business value, LLMs need to be able to pull data sets from across an organization: think website data, inventory data, CRM data, ERP data, and payments data. Businesses often lack a complete customer picture within a single pane of glass, and for LLMs to work— particularly if their use case is delivering a hyper-personalized customer experience— a unified data lake is a prerequisite. Want your company’s LLM to know your customer’s name, purchase history, credit card number, address, page visits, engagement patterns — all necessary data sets to deliver a superlative customer experience? More likely than not, significant data engineering investments will be required. And by the way, once said data lake is created, organizations still have to contend with Challenge #1: the data lake’s integrations will need to be maintained and tended in perpetuity, lest your data lake run dry.
Challenge #3: Business Strategies Shift.
Off-the-shelf LLMs produce responses that are flirtatious, fun, and witty. This is precisely what has allowed ChatGPT to capture the hearts and minds of consumers, and such consumer revenues comprise 75%+ of OpenAI’s business at present. This is also precisely why off-the-shelf LLMs are so poorly suited to deliver business outcomes: they allow conversations with consumers to meander, they go off script, they concoct stories, and they almost always fail to drive consumers towards the desired business outcome. Query an online retailer’s LLM about an out of stock product, and you might be surprised to have the LLM refer you to said retailer’s arch-competitor. This is an unforced error, and a simple one which will soon be addressed. More complex errors that naturally come from evolving approaches to nuanced business strategies such as prioritizing certain products with certain customer cohorts, seasonal initiatives, or brand compliance norms will be much more difficult to solve without verticalization.
Challenge #4: Don’t get hacked.
Congratulations on making it this far. You’ve deployed a state-of-the-art custom LLM, tapped your crystalline, artifact-free data lake, and managed to incorporate dynamic business logic to drive this quarter’s desired outcomes… and you’ve just been hacked. Custom LLMs, and the trove of PII (Personally Identifiable Information) that they necessarily incorporate to deliver value, present an unprecedentedly rich target for cyber criminals. Defending an LLM’s attack area is an endeavor only the most sophisticated GenAI companies have figured out how to address, and like the other GTM challenges we’ve surveyed, will require constant investment to remain one step ahead of bad actors.
Don’t Choke.
Generative AI, like any platform shift, will yield a fresh set of winners and losers, many of them unexpected. When “Software is Eating the World” was coined by Marc Andressen a little over two years after the 2008 dotcom crash, public markets still smarting from the pain were valuing Apple at a paltry 14x PE multiple (today Apple trades at 40x). The Generative AI market and its leaders today will almost certainly face tough times at some point in the future, and almost certainly new, unheard of, world-eating GenAI companies will emerge.
I myself am betting on Vertical AI.